About This Project
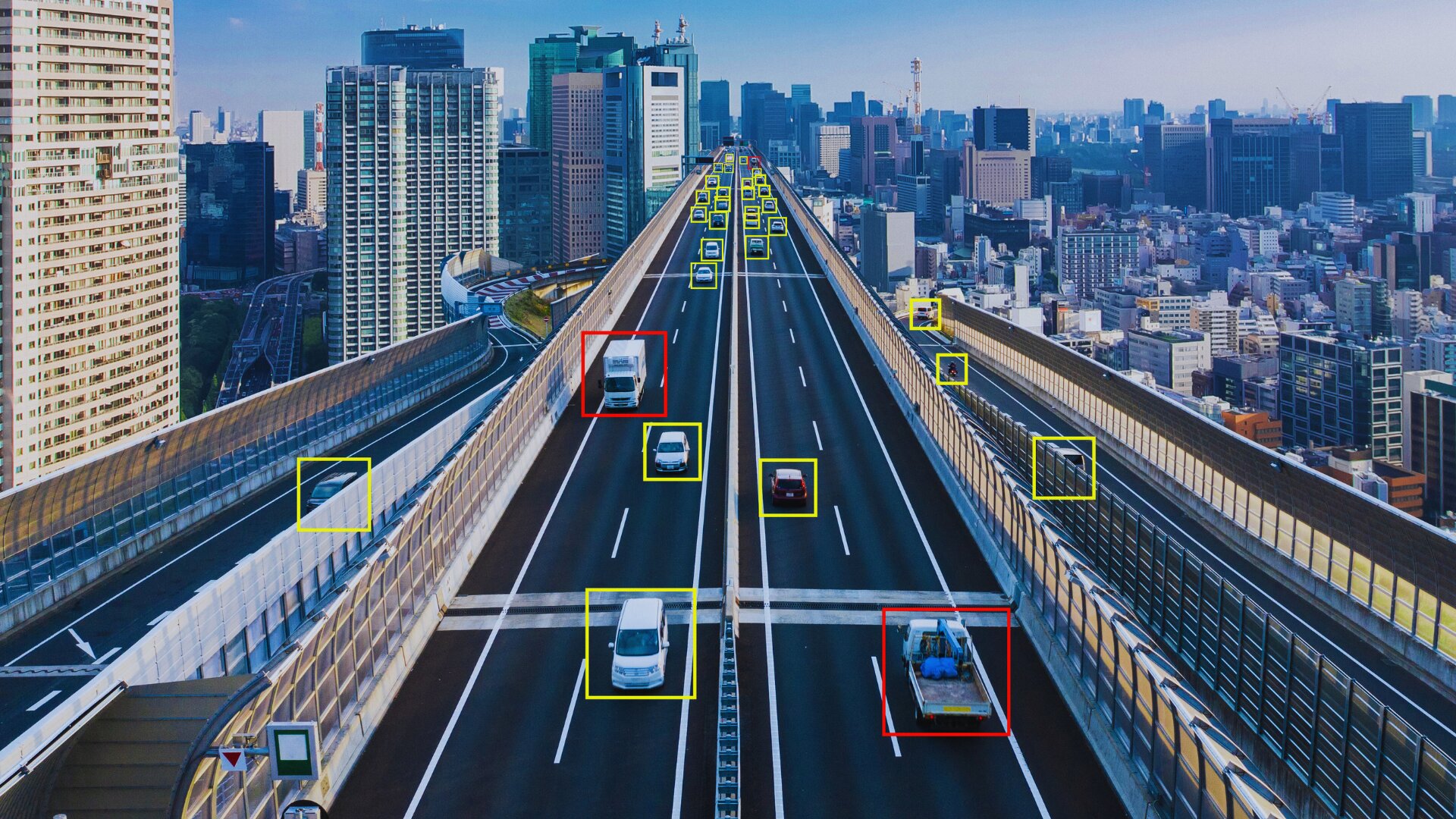
GossipRSU is a decentralized federated learning framework designed to optimize model training across roadside units (RSUs) in intelligent transportation systems (ITS). Unlike traditional FL approaches that focus on vehicle-side training, GossipRSU categorizes RSUs into two groups: Corridor RSUs (C-RSUs), which collect IID data from vehicles along the same road segment, and Crossroad RSUs (X-RSUs), which gather non-IID data from intersections and diverse road networks. By leveraging gossip learning, RSUs exchange model updates in a fully decentralized manner, eliminating the need for a central aggregator and reducing communication bottlenecks. GossipRSU dynamically adapts its learning strategy based on data characteristics, allowing C-RSUs to use traditional FL methods while X-RSUs employ personalized or meta-learning approaches. This decentralized and adaptive design enhances model convergence, scalability, and robustness, making GossipRSU a promising solution for real-world ITS deployments.